Vikram Shenoy, a Ph. D. student at Nanyang Technological University in Singapore won the annual competition for the best oral presentation and paper at the IEEE Systems, Man, and Cybernetics Society Conference. The paper’s title is “An Iterative Optimization Technique for Robust Channel Selection in Motor Imagery based Brain Computer Interface” (co-author Vinod Achutavarrier Prasad).
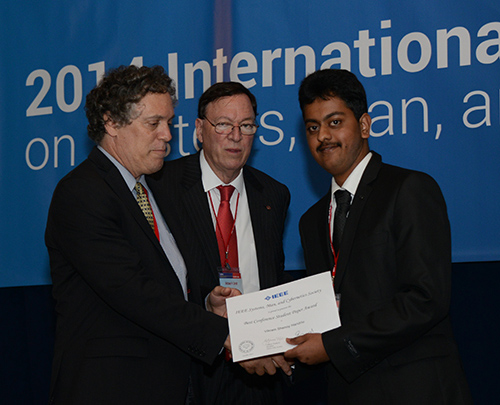
Brain-Computer Interface (BCI) is an interesting research area which opens up the avenue for decoding the human intention, which was in the realm of science fiction so far. An increasing number of patients every year with stroke, neuromuscular disorders like Amyotrophic Lateral Sclerosis (ALS), cerebral palsy etc. requires an effective neurorehabilitation strategy for which, BCI could be a viable option. BCI provides a direct communication and control pathway between brain and computer/machine bypassing the conventional pathway of nerves and muscles. Electroencephalography (EEG) is the most commonly used brain signal acquisition technique in BCI systems. The use of motor imagery (imagination of movement of limbs) patterns in EEG-based BCI has been proven as an effective method to translate the user’s movement intention into commands for controlling external devices like robotic arm which assists in neurorehabilitation.
Conventional EEG headsets come with variable number of sensing electrodes called channels (as sparse as 16 channels to as dense as 256 channels). The use of fewer channels results in computational efficiency, but reveals very limited information about the brain activity. Meanwhile, large numbers of channels uncover more information about the brain signal but results in increased computation and experimental preparation time which is not advisable in real-time BCI applications. To strike the balance between the two, it is necessary to optimize the number of EEG channels being used. In the work presented in this paper, authors use apriori information of the motor imagery task to propose an iterative method for selecting the most relevant channels. The authors make use of publicly available BCI competition datasets with 118 channels (dense) and 22 channels (sparse) to validate whether the algorithm is invariant to number of channels being used. The proposed method results in better accuracy of classifying the movement imagination between right hand and left hand compared to state-of-the-art methods with a significant reduction in the number of channels. The authors have looked into another interesting aspect of handling subject-variability, which is hardly explored in the literature. Each individual has different head geometry but the EEG headsets come with a standard size. This makes it little difficult to generalize the signal information from different parts of the brain scalp. The proposed method addresses this variability between different subjects based on frequently selected channels across subjects thereby revealing the significance of different channels. This will aid in substantially reducing the preparation time when performing multiple session BCI experiments for a larger pool of subjects, especially when using high dense EEG headsets. Having demonstrated the good classification accuracy with far lesser computation time, the authors believe that the proposed method might prove beneficial in online motor imagery BCI experiments.
Vikram Shenoy’s research is part of a program lead by Professor Vinod A Prasad, Nanyang Technological University, in collaboration withand Dr Guan Cuntai, Institute for Infocomm Research, A*STAR, Singapore. Their work focuses on Robust and accurate signal processing techniques for robust and accurate Brain-Computer Interfaces (BCI.) This includes:
- Robust and accurate motor imagery classification algorithms
- Decoding limb movement kinematics from Electroencephalogram-based BCI
- BCI-based neurofeedback games for enhancing cognition skills.
The group proposed robust and accurate signal processing algorithms to extract discriminative brain activation patterns during hand, foot and tongue in an EEG based BCI system. The group further investigated the intra subject and inter subject spectral variability of discriminative motor patterns in EEG and have proposed algorithms to effectively track the varying patterns. The non-stationary features of EEG signals were adaptively estimated in the proposed algorithms and the results achieved indicate that the proposed approach outperforms the state-of-art methods in terms of classification accuracy and highlights the necessity of efficient frequency band selection techniques in real time MI-BCI applications. They also contributed in another area of movement control BCIs that aims to decode hand movement kinematics from non-invasive scalp recordings. Moreover, they introduced algorithms for demonstrating the presence of movement parameter information in specific space, frequency and time locations of EEG and have developed feature extraction tools to detect them. Significant contributions were made in binary classification of hand movement speed and direction. The proposed algorithms were modified further to achieve multiclass classification of direction and continuous reconstruction of hand movement speed and trajectory. They developed BCI-based Computer games to enhance attention (concentration) and memory of children with attention-deficit hyperactivity disorder (ADHD). Such children exhibit lack of attention, change in the behavioural mood, hyperactivity and impulsivity. Worldwide, ADHD is common with an estimated prevalence rate of 5.3%. In Singapore, ADHD ranks as the third highest cause of disease burden in youths below the age of 14. Medications often cause significant side effects including poor appetite and physical growth suppression, and have only limited impact on ADHD treatment. Alternative methodologies to train children for enhancing their concentration (attention) skills need to be developed to complement conventional pharmacological treatment. The Our research team developed a brain wave (EEG) driven computer game that can be used by children suffering from ADHD to boost their concentration abilities. Brain signal corresponding to concentration of subjects is used to control the game, which in turn helped to improve the concentration abilities of ADHD children by playing the game in a relaxed mindset, without the need of undergoing complex behavioural treatment procedures. TheyWe developed a BCI system comprising of EEG data acquisition, EEG signal processing methods and computer game which can be controlled with the concentration data acquired from children.
Dr. Guan Cuntai of the Institute for Infocomm Research, A*STAR, Singapore collaborates with the team whose members are Postdoctoral Fellows Dr. Kavitha P. Thomas, Dr. Smitha K. G, and Research Staff, Ms. Neethu Robinson. The team leader, Vinod A Prasad received his Ph. D. degree from School of Computer Engineering, Nanyang Technological University (NTU), Singapore, in 2004.
From September 2000 to September 2002, he was a Lecturer in Singapore Polytechnic, Singapore. He joined NTU as a Lecturer in the School of Computer Engineering in September 2002 where he is currently a tenured Associate Professor. He has published over 180 papers in refereed international journals and conferences, supervised and graduated 8 Ph. D.’s He is a Senior Member of IEEE, Associate Editor of IEEE Transactions on Human-Machine Systems, Associate Editor of Springer Journal Circuits, Systems, and Signal Processing Journal (Springer), and Technical Committee Co-Chair of Brain-Machine Interface Systems of IEEE Systems, Man & Cybernetics Society. He has won the Nanyang Award for Excellence in Teaching in 2009, the highest recognition conferred by NTU to individual faculty for teaching.